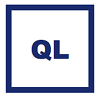
Qardian Labs Founder inspired by grandmother’s undetected heart problems
By Tom Ballard, Chief Alliance Officer, PYA
“I’ve always been passionate about healthcare . . . finding new solutions to improve outcomes,” Sofia Tomov told us in a recent interview. “I developed an algorithm in middle school that found genetic mutations about adverse drug interactions.”
The winner of last fall’s “Boyd Venture Challenge” at the University of Tennessee and the recent “Scots Innovation Challenge,” hosted by Maryville College, also has her own start-up named Qardian Labs. Launching a company and winning competitions is not that unusual for a college student like Tomov with one exception: she’s a University of Tennessee sophomore but not yet 16 years old.
Just for good measure, she also captured a first place award and $3,000 in the “Translational Medical Science” portion of the 2018 “Intel International Science and Engineering Fair” in Pittsburgh.
How did the budding entrepreneur get interested in healthcare?
“My grandmother collapsed at my 10th birthday party,” Tomov explained. “No one in the family knew that she had heart problems.”
With her naturally inquisitive nature, she decided to find a better way to help with early and reliable diagnosis of heart disease. After all, Tomov learned that heart disease kills 17 million people a year, and one in three are misdiagnosed.
Why?
“The main tests that doctors are using are not computer-based,” Tomov explained. She settled on data analytics as the best approach, using what she describes as “applying deep learning in a novel way,”
So, what’s different about what Tomov is providing to enhance what your Primary Care Physician would normally do? The healthcare professional looks at a patient’s test results and other information – age, sex, chest pain type, resting blood pressure, cholesterol level, resting blood sugar reading, resting electrocardiographic results, maximum heart rate achieved and several other factors.
When considered in isolation for just one patient, it becomes more challenging for a doctor to discern patters. The Qardian Labs algorithm analyzes relationships between numbers across a population base and discovers patterns that might not be seen by the doctor.
It does not provide a diagnosis; it is only a tool to help the healthcare professional do that.
We asked Tomov to explain of how she has validated the algorithm.
“I tested my algorithm using the University of California Irvine machine learning repository dataset with real patient information and outcomes,” she explained. “I divided the dataset into two-thirds training data and one-third test data, a ratio recommended by data analysis experts such as Andrew Ng, where the algorithm learns from training data and applies itself to unfamiliar test data.”
On the test dataset, Tomov says the algorithm correctly diagnosed 99% of the patients without previous exposure to the data.
“I further validated the percent accuracy by conducting statistical tests like Matthews Correlation Coefficient, precision, recall, and F1 score,” she added. “These metrics all measure classification performance specific to prediction outcomes such as true positive, true negative, false positive, and false negative. This helps ensure that the performance evaluation is fair and independent of potential dataset bias. Because my software achieves nearly optimal statistical measurements, the results are indicative of its ability to apply itself to a wide variety of data in a clinical setting.”
Tomov continues to further refine the algorithm in a variety of ways. She has linked-up with Bruce Ramshaw, until recently the Chief of Surgery at the University of Tennessee Medical Center (UTMC) and Founder of CQ Insights, a healthcare data analytics company. She has commitments from a UTMC cardiology group and another practice in Memphis to help.
Tomov sees Qardian Labs “go-to-market” approach as Software as a Service and hopes to eventually build a strategic partnership with one of the big Electronic Medical Records providers.
Like what you've read?
Forward to a friend!